Evolution of Sex Determination Networks

Recent evidence indicates that an increase in the complexity of interactions has played an important role in gene network evolution. Sex determination mechanisms offer a good model for studying gene network evolution because, among other reasons, they evolve rapidly. We are using a modelling approach to study network evolution, and are working on a hierarchical model which integrates two previously separate modelling techniques, from population genetics on the one hand, and network dynamics on the other. The theoretical model has been used to investigate the early evolution of sex determination networks. Following from a hypothesis proposed by A.S.Wilkins, we assume that sex determination networks have evolved in a retrograde manner from bottom to top. Starting from a simplest possible ancestral system, based on a single locus, we investigate how more complex systems, involving more loci, could have evolved. We are also investigating evolution between species for which sex determination is (at least partially) understood: D. melanogaster, medfly, honeybee, domstic housefly, and silkworm.
On the Evolution of Gene Networks
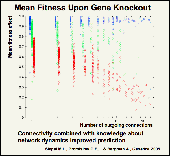
We aim at understanding how evolution affects interacting genes of gene networks.The relationship between the topology of a biological network and its functional or evolutionary properties has attracted much recent interest. It has been suggested that most, if not all, biological networks are 'scale free.' That is, their connections follow power-law distributions, such that there are very few nodes with very many connections and vice versa. The number of target genes of known transcriptional regulators in the yeast, Saccharomyces cerevisiae, appears to follow such a distribution, as do other networks, such as the yeast network of protein-protein interactions. These findings have inspired attempts to draw biological inferences from general properties associated with scale-free network topology. One often cited general property is that, when compromised; highly connected nodes will tend to have a larger effect on network function than sparsely connected nodes. For example, more highly connected proteins are more likely to be lethal when knocked out. However, the correlation between lethality and connectivity is relatively weak, and some highly connected proteins can be removed without noticeable phenotypic effect. Similarly, network topology only weakly predicts the response of gene expression to environmental perturbations. Evolutionary simulations of gene- regulatory networks suggest that such weak or nonexistent correlations are to be expected, and are likely not due to inadequacy of experimental data. We argue that 'top-down' inferences of biological properties based on simple measures of network topology are of limited utility, and our simulation results suggesting that much more detailed information about a gene's location in a regulatory network, as well as dynamic gene-expression data, are needed to make more meaningful functional and evolutionary predictions. Specifically, we find in our simulations that: 1) the relationship between a gene's connectivity and its fitness effect upon knockout depends on its equilibrium expression level; 2) correlation between connectivity and genetic variation is virtually nonexistent, yet upon independent evolution of networks with identical topologies, some nodes exhibit consistently low or high polymorphism; and 3) certain genes show low polymorphism yet highdivergence among independent evolutionary runs. This latter pattern is generally taken as a signature of positive selection, but in our simulations its cause is often neutral co-evolution of regulatory inputs to the same gene.